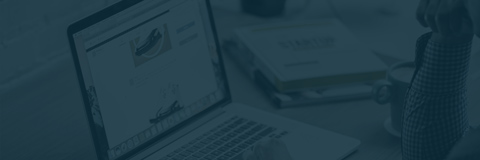
PyCon 2017 (Part 2!) Talk: “Double Click: Continue Building Better CLIs” Summarized by Kévin Barralon This PyCon Canada talk was a presentation of Click, a Python package that allows you to create CLIs (Command-Line Interfaces) with only a few lines of code. Click is simple and easy to use, in that the configuration of commands […]